There is no such thing as universal data quality. There, I’ve said it.
When it comes to product information, we have become almost obsessed with assessing quality. In practice, there are two fundamental flows in which packaged goods manufacturers measure and assess data quality. First, the quality of our data depends largely on the context – what are we going to use the data for in the first place? Second, we tend to confuse data completeness (computer says “all fields are filled”) with data quality. Just because a value is entered and the system reports 100% completeness, that doesn’t mean that the data is correct or valid.
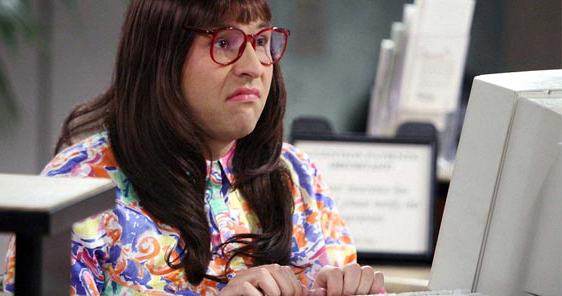
Quality is in the eye of the beholder
First things first; what does ‘quality’ actually mean? Take a simple product like glue. In many people’s perception, the quality of superglue is superior to that of a glue stick. After all, it does a better job of quickly and firmly gluing objects together.
If you’re a kindergarten teacher, however, you will probably prefer a glue stick – for reasons that I shall not elaborate on. The same goes for many products. A Ferrari is considered to be a higher quality car than a Dacia Duster. But if I need to transport a family of seven, I certainly know which car I’d use.
The quality of anything – products and data alike – entirely depends on what you want to do with it. It’s impossible to assess the quality of data without knowing what the purpose of this data is. If you are a manufacturer of glue, you will need different types of information for consumer retail channels than for professional products, where ETIM plays an important role.
Let’s look at another example. If you are a building materials manufacturer, you will need different types of information depending on the channel; for the professional channel in the different commercial stages, starting with architects and advisors, to professional builders, installers, building maintenance and the users/residents. But in do-it-yourself stores, the information needs are completely different. As a result, the quality of data for one product means something entirely different for one channel than for the other.
Dogma
You may ask: what’s the big deal? Why is this even a problem? Well, the thing is: most systems don’t take context into account when assessing data quality. What’s more, they confuse ‘quality’ with ‘100% existence of 100% filled fields’.
Ted Friedman from Gartner defines six objective dimensions data quality:
- Existence (Are data available in the first place?)
- Validity (Do data fall within an acceptable range or domain?)
- Completeness (are all the data necessary to meet the current and future business information demand available?)
- Accuracy (Do the data describe the properties of the object they are meant to model?)
- Integrity (Are the relationships between data elements and across data sets complete?)
- Consistency (Do data stored in multiple locations contain the same values?)
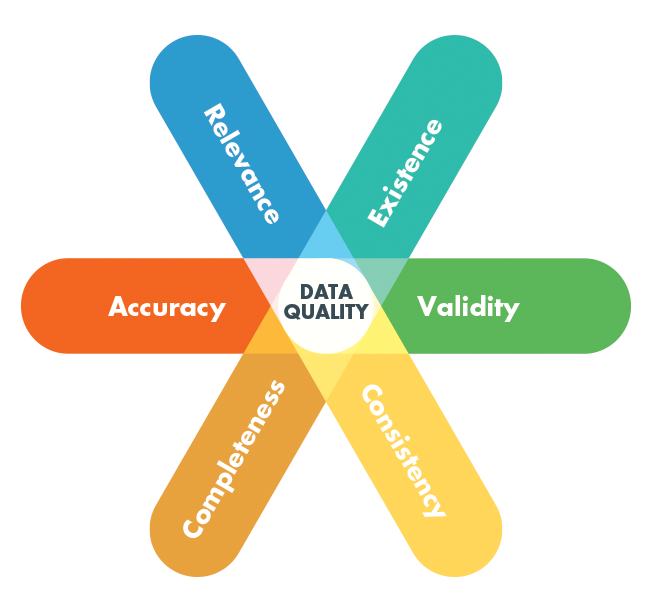
Friedman also defines, next to these objective dimensions, six subjective data quality dimensions: Timeliness, Relevance, Interpretability, Understandability, Objectivity and Trust.
Many PIM, DAM and PLM systems only assess the quality of data based on the percentage fields that contain data (another word for existence, criteria #1) and some might include a validity check (#2). That doesn’t say anything about what really matters, like consistency, accuracy, integrity and even completeness; let alone subjective dimensions of data quality such as delivering the right and relevant information to the right channel at the right time.
Dummy data
If you confuse data quality with 100% data existence – and this happens more than most manufacturers would like to admit – you risk encouraging employees to enter data for the sake of entering data. This is what a lot of us tend to do when filling in forms on the internet; sometimes we enter dummy data, just to be done with it. If you are buying a product online and a vendor asks you to enter personal data that is totally irrelevant for the purchasing or delivery process, you are probably inclined to just enter fake data and hope to get away with it.
Chances are that your employees are doing that all the time when they enter product data into your systems. And they are probably even entitled to do so because the data (or their purpose) is not yet known. Imagine R&D engineers who need to enter a description of a product that is in the early stages of development. If they are asked to enter the colour of the product and it’s too early to tell, they will probably enter a dummy variable, like ‘black’, as long as the system doesn’t protest. In later stages of the Strategy-to-Market process, when the product colour has been chosen, it may conflict with the dummy data that was entered originally – simply resulting in incorrect data.
So, what is the best way to deal with data quality?
First, make sure you know the purpose of the data. This is what we call context validation. You need to know the general guidelines for product data, but also rules and guidelines for each product category, geographic region, sector and channel (such as retail, e-commerce or GS1).
Only when you know the market touchpoints and canals that are relevant to a specific product, then you will be able to determine criteria for assessing the quality of your data.
In our content syndication engine, data is validated based on the context for every individual product. In other words: data is approved depending on what you need them for. Just as the quality of the glue depends on whether you will use it in construction or in a kindergarten.
It all depends on the context.